AI for Predictive Maintenance Applications in Manufacturing
Dr. Evangelo Damigos; PhD | Head of Digital Futures Research Desk
- AI
- Sustainable Growth and Tech Trends
- Emerging Technologies
Publication | Update: Sep 2020

Predictive maintenance (PdM) based on data-driven methods has become the most effective solution to address smart manufacturing and industrial big data, especially for performing health perception (e.g., fault diagnosis and remaining life assessment).
Taking advantage of artificial intelligence capabilities, such as of recognizing temperature, vibration, and other factors from sensors pre-built into machinery and vehicles, business leaders adopt predictive and preventative maintenance applications.
According to Pamela Bump, Managing Editor at Emerj, while predictive maintenance allows manufacturers to attempt to predict how long a piece of machinery will last, preventative maintenance involves repairing the machinery to keep it working longer.
With the digitization of many manufacturing plants and the reported costs of reactive maintenance needed to fix machinery in mind, Emerj has put together a list of five companies that claim to offer software using AI for predictive maintenance.
These companies offer software for use in two applications:
- Asset Performance: The company’s primary goal is using sensors or other data to determine and notify manufacturers when a piece of machinery is broken or not working.
- CMMS Systems: Systems that both monitor asset performance and allow users to assign and track repair work orders.
According to Daniel Faggella, Head of Research at Emerj, upkeep on physical equipment is expensive and time-consuming. In addition to the cost of human diagnostic efforts by trained professionals, equipment downtime can have downstream productivity impacts.
When a piece of manufacturing equipment (such as a drill or a lathe) is shut down for maintenance, the in-process inventory being fed to that machine must halt or be routed elsewhere. Backed up in-process inventory can create unpredictable downstream effects for other equipment, for transportation, and for the labor forces operating them – not to mention a potential failure to meet customer delivery expectations and deadlines.
Because the expense and impact of a breakdown or malfunction of equipment is so significant, manufacturing firms business must have consistent regiments of maintenance and upkeep in order to limit productivity disruption while also keeping a close pulse on the functioning of it’s most important equipment.
The potential for artificial intelligence to reduce downtime while also improving overall maintenance and monitoring of equipment is significant. Reducing risk and improving throughput (revenue) are important to any manufacturing operation.
According to ReadITQuik review, businesses in the manufacturing space are looking at ways to optimize processes, both in terms of efficiency and costs. The use of data-backed “intelligent” algorithms is a great way to make that happen. As a result, businesses are turning to AI applications, one of the most beneficial ones being predictive maintenance.
Predictive maintenance is thus the core of manufacturing innovation and involves rethinking and optimizing the entire maintenance strategy as a whole from top to bottom.
Here is how companies must go about embracing this new technique:
1. Understand the Need: The first step in moving toward predictive maintenance is to understand pain points (namely drivers of costs, waste, or inefficiency) and identify the best use case for your business.
2. Get Data: Of course, the proliferation of IoT plays a large role in predictive maintenance, especially with cheap sensors and data storage combined with more powerful data processing that has made the technology accessible. But, there are other data sources out there, which might include data from programmable controllers, manufacturing execution systems, building management systems, manual data from human inspection, static data such as manufacturer service recommendations for each asset, external data from APIs, like weather, that could impact equipment conditions or wear, geographical data, equipment usage history data, and parts composition.
3.. Explore and Clean Data: After identifying relevant data sets, it’s time to dig in. Ensure you really understand all the data you’re dealing with and that you know what all of the variables mean, what is being measured, and where all the data is coming from.
4. Enrich Data: Manipulating data at this stage means adding more features and connecting them in meaningful ways so that each data set, or data from multiple sensors, can be taken as a whole instead of in parts.
5. Get Predictive: It is this combination of a variety of sources and data types that allows for the most robust and accurate predictive models. The more sources and types of data available, the better the complete picture of a particular asset and the better the prediction.
6. Visualization: Visualization is an important tool in predictive maintenance as it often closes the feedback loop, allowing maintenance managers and staff to see the outputs of predictive models and direct their attention accordingly. Robust data science or data team tools today allow maintenance managers and staff on-the-ground to easily access and digest outputs in a familiar format so that the entire team, from analysts to technicians receive the same feedback.
7. Deploy: Deploying a predictive maintenance model into production means working with real-time data, but to iterate and deploy means providing visual real-time dashboards for on-the-ground maintenance teams. For some use cases, feedback can be integrated directly into the predictive maintenance process, requiring no or little human interaction.
Overall, predictive maintenance shows huge promise for the future with its potential cost savings, new revenue channels, and a high degree of automation leading to less dependence on human resources.
Read More on 5 Use Cases: https://emerj.com/ai-sector-overviews/ai-for-predictive-maintenance-applications-in-industry-examining-5-use-cases/
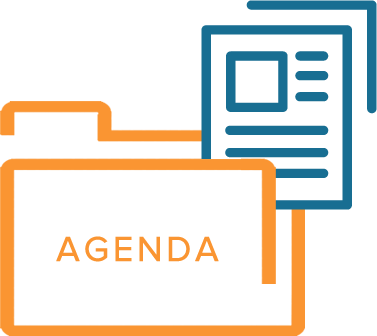

HTML



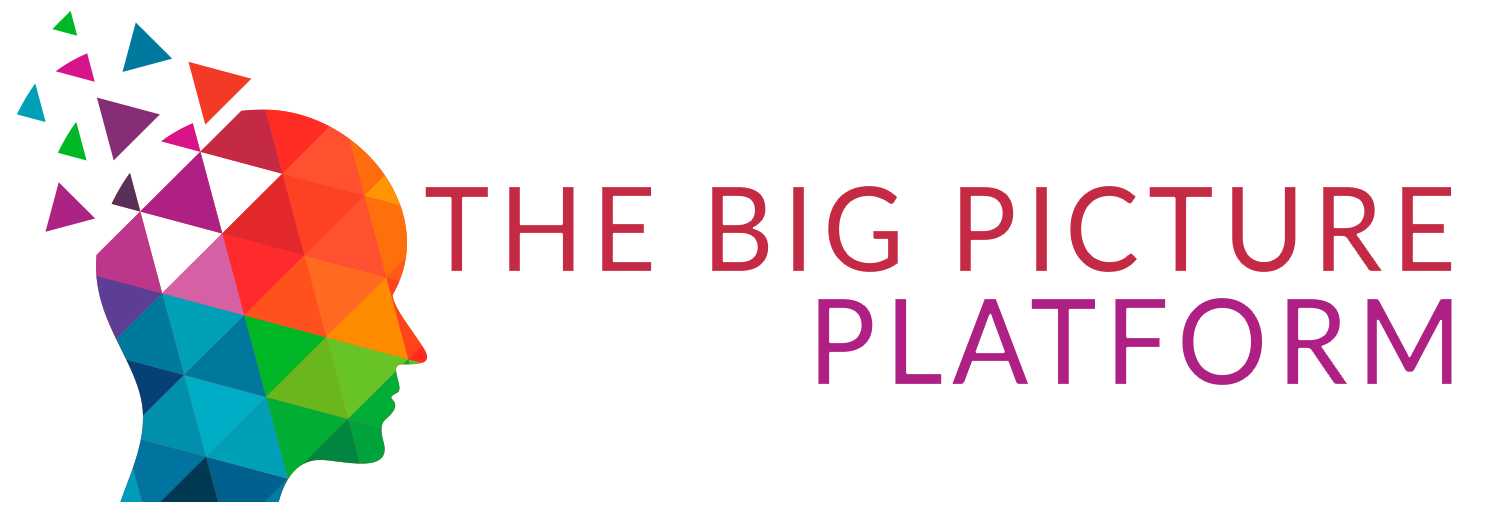
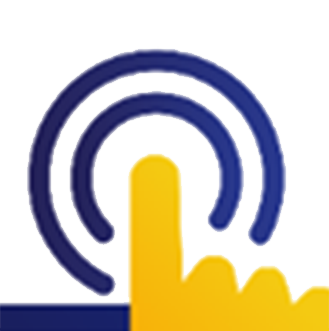
Objectives and Study Scope
This study has assimilated knowledge and insight from business and subject-matter experts, and from a broad spectrum of market initiatives. Building on this research, the objectives of this market research report is to provide actionable intelligence on opportunities alongside the market size of various segments, as well as fact-based information on key factors influencing the market- growth drivers, industry-specific challenges and other critical issues in terms of detailed analysis and impact.
The report in its entirety provides a comprehensive overview of the current global condition, as well as notable opportunities and challenges.
The analysis reflects market size, latest trends, growth drivers, threats, opportunities, as well as key market segments. The study addresses market dynamics in several geographic segments along with market analysis for the current market environment and future scenario over the forecast period.
The report also segments the market into various categories based on the product, end user, application, type, and region.
The report also studies various growth drivers and restraints impacting the market, plus a comprehensive market and vendor landscape in addition to a SWOT analysis of the key players.
This analysis also examines the competitive landscape within each market. Market factors are assessed by examining barriers to entry and market opportunities. Strategies adopted by key players including recent developments, new product launches, merger and acquisitions, and other insightful updates are provided.
Research Process & Methodology

We leverage extensive primary research, our contact database, knowledge of companies and industry relationships, patent and academic journal searches, and Institutes and University associate links to frame a strong visibility in the markets and technologies we cover.
We draw on available data sources and methods to profile developments. We use computerised data mining methods and analytical techniques, including cluster and regression modelling, to identify patterns from publicly available online information on enterprise web sites.
Historical, qualitative and quantitative information is obtained principally from confidential and proprietary sources, professional network, annual reports, investor relationship presentations, and expert interviews, about key factors, such as recent trends in industry performance and identify factors underlying those trends - drivers, restraints, opportunities, and challenges influencing the growth of the market, for both, the supply and demand sides.
In addition to our own desk research, various secondary sources, such as Hoovers, Dun & Bradstreet, Bloomberg BusinessWeek, Statista, are referred to identify key players in the industry, supply chain and market size, percentage shares, splits, and breakdowns into segments and subsegments with respect to individual growth trends, prospects, and contribution to the total market.
Research Portfolio Sources:
Global Business Reviews, Research Papers, Commentary & Strategy Reports
M&A and Risk Management | Regulation
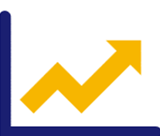
The future outlook “forecast” is based on a set of statistical methods such as regression analysis, industry specific drivers as well as analyst evaluations, as well as analysis of the trends that influence economic outcomes and business decision making.
The Global Economic Model is covering the political environment, the macroeconomic environment, market opportunities, policy towards free enterprise and competition, policy towards foreign investment, foreign trade and exchange controls, taxes,
financing, the labour market and infrastructure.
We aim update our market forecast to include the latest market developments and trends.
Review of independent forecasts for the main macroeconomic variables by the following organizations provide a holistic overview of the range of alternative opinions:
As a result, the reported forecasts derive from different forecasters and may not represent the view of any one forecaster over the whole of the forecast period. These projections provide an indication of what is, in our view most likely to happen, not what it will definitely happen.
Short- and medium-term forecasts are based on a “demand-side” forecasting framework, under the assumption that supply adjusts to meet demand either directly through changes in output or through the depletion of inventories.
Long-term projections rely on a supply-side framework, in which output is determined by the availability of labour and capital equipment and the growth in productivity.
Long-term growth prospects, are impacted by factors including the workforce capabilities, the openness of the economy to trade, the legal framework, fiscal policy, the degree of government regulation.
Direct contribution to GDP
The method for calculating the direct contribution of an industry to GDP, is to measure its ‘gross value added’ (GVA); that is, to calculate the difference between the industry’s total pretax revenue and its total boughtin costs (costs excluding wages and salaries).
Forecasts of GDP growth: GDP = CN+IN+GS+NEX
GDP growth estimates take into account:

Market Quantification
All relevant markets are quantified utilizing revenue figures for the forecast period. The Compound Annual Growth Rate (CAGR) within each segment is used to measure growth and to extrapolate data when figures are not publicly available.
Revenues
Our market segments reflect major categories and subcategories of the global market, followed by an analysis of statistical data covering national spending and international trade relations and patterns. Market values reflect revenues paid by the final customer / end user to vendors and service providers either directly or through distribution channels, excluding VAT. Local currencies are converted to USD using the yearly average exchange rates of local currencies to the USD for the respective year as provided by the IMF World Economic Outlook Database.
Industry Life Cycle Market Phase
Market phase is determined using factors in the Industry Life Cycle model. The adapted market phase definitions are as follows:
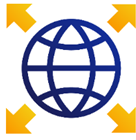
The Global Economic Model
The Global Economic Model brings together macroeconomic and sectoral forecasts for quantifying the key relationships.
The model is a hybrid statistical model that uses macroeconomic variables and inter-industry linkages to forecast sectoral output. The model is used to forecast not just output, but prices, wages, employment and investment. The principal variables driving the industry model are the components of final demand, which directly or indirectly determine the demand facing each industry. However, other macroeconomic assumptions — in particular exchange rates, as well as world commodity prices — also enter into the equation, as well as other industry specific factors that have been or are expected to impact.
Forecasts of GDP growth per capita based on these factors can then be combined with demographic projections to give forecasts for overall GDP growth.
Wherever possible, publicly available data from official sources are used for the latest available year. Qualitative indicators are normalised (on the basis of: Normalised x = (x - Min(x)) / (Max(x) - Min(x)) where Min(x) and Max(x) are, the lowest and highest values for any given indicator respectively) and then aggregated across categories to enable an overall comparison. The normalised value is then transformed into a positive number on a scale of 0 to 100. The weighting assigned to each indicator can be changed to reflect different assumptions about their relative importance.

The principal explanatory variable in each industry’s output equation is the Total Demand variable, encompassing exogenous macroeconomic assumptions, consumer spending and investment, and intermediate demand for goods and services by sectors of the economy for use as inputs in the production of their own goods and services.
Elasticities
Elasticity measures the response of one economic variable to a change in another economic variable, whether the good or service is demanded as an input into a final product or whether it is the final product, and provides insight into the proportional impact of different economic actions and policy decisions.
Demand elasticities measure the change in the quantity demanded of a particular good or service as a result of changes to other economic variables, such as its own price, the price of competing or complementary goods and services, income levels, taxes.
Demand elasticities can be influenced by several factors. Each of these factors, along with the specific characteristics of the product, will interact to determine its overall responsiveness of demand to changes in prices and incomes.
The individual characteristics of a good or service will have an impact, but there are also a number of general factors that will typically affect the sensitivity of demand, such as the availability of substitutes, whereby the elasticity is typically higher the greater the number of available substitutes, as consumers can easily switch between different products.
The degree of necessity. Luxury products and habit forming ones, typically have a higher elasticity.
Proportion of the budget consumed by the item. Products that consume a large portion of the
consumer’s budget tend to have greater elasticity.
Elasticities tend to be greater over the long run because consumers have more time to adjust their behaviour.
Finally, if the product or service is an input into a final product then the price elasticity will depend on the price elasticity of the final product, its cost share in the production costs, and the availability of substitutes for that good or service.
Prices
Prices are also forecast using an input-output framework. Input costs have two components; labour costs are driven by wages, while intermediate costs are computed as an input-output weighted aggregate of input sectors’ prices. Employment is a function of output and real sectoral wages, that are forecast as a function of whole economy growth in wages. Investment is forecast as a function of output and aggregate level business investment.