A Design Thinking Mindset for Data Science
Dr. Evangelo Damigos; PhD | Head of Digital Futures Research Desk
- Connected Intelligence
Publication | Update: Mar 2020

A successful data science project begins with strategic question and selection of the area of research. Design Thinking describes a strategic selection process as balancing the technical feasibility, desirability, and business viability. This mindset forces design solutions to consider the human elements to a solution in conjunction with the business and technical aspects, which is an area that is often overlooked. The following proposes a similar venn diagram for the selection of a data science research area to aid in increasing the chance for success in a data science project: the intersection of technical feasibility, business impact and data availability.

Another important aspect of the ideation phase is the framing and scoping of the problem, including determining hypothesis, important questions, and goals of the research. In design thinking, since the designer is often not the subject matter expert, designers conduct primary and secondary research nearly from the second the project is assigned to start developing an understanding of the problem space. Often during these early stages, designers are pleasantly surprised to gather deeper insights than expected. A simple question of asking to be walked through the process may illuminate pains and problems even if the designer or researcher isn’t explicitly asking for them.
Data scientists can apply the same techniques to help familiarize themselves with both the area of interest, get to know the stakeholders, and uncover insights that will assist in framing their research process. Applying this mindset could look like the beginning stages of a data science research project including ample upfront research. Examples of activities to perform include:
- Informational interviews with other business units and teams
- Diagramming processes and concepts to test understanding
- Accessing user testimonials and support tickets, when applicable
Exploratory Analysis
Entering the exploratory phase of a project can seem disorganized, making it difficult to come up with ideas and lead to creative burnout. Design thinking approaches this phase in the design process by creating process diagrams and frameworks to organize key learnings, identify areas of further interest, and communicate decisions to outside stakeholders. Examples of exploratory tools used in design thinking include As-Is Journey Maps and Customer Journeys. Similar tools, or simply drawing out a concept of the research area can help data scientists organize and strategize their next steps in the exploratory phase.
Brainstorming and creativity in analysis techniques are also a key part of the exploratory phase. As described previously in this paper, data scientists can experience frustration when they are unable to think of new analysis questions in attempts to uncover an interesting area for further research. An important theory from design thinking that can be borrowed for data science methods is the idea of quantity over quantity in the early stages of a project. Instead of limiting ideas from the beginning, start by writing out 100 potential questions or queries that can be made of the data, no matter how absurd or un-useful, then bundle these ideas into themes and prioritize those by easiness versus potential impact.
Modeling, Prototyping and Deeper Analysis
The design thinking process benefits from rapid iteration and targeted feedback from relevant stakeholders, allowing a larger range of possible solutions to be considered in the selection process. This strategy aims to avoid personal biases and the selection of the first idea when a better idea may have come along down the road. The data science process can benefit from a similar strategy to help increase the creativity and options considered, while avoiding pigeon-holing a solution based on the first idea that was tried.
Gathering feedback from relevant stakeholders also has benefits when it comes to communication and buy-in. For example, designers use feedback not only as a method of gathering input on potential solutions, but also for learning how to best position solutions and share findings with specific audiences. Similarly, data scientists often struggle with ensuring the project’s results are relevant to stakeholders, and if they are, how to communicate it. Thus, frequent and intentional feedback throughout the process of modeling and creating deeper analysis could improve these weaknesses.
Presenting Findings and Models
Most research processes gather a plethora of data and insights, typically more than is relevant and valuable to an audience. As a result, the results often need to be distilled down to what is really important. Design thinking approaches this step by framing the insights and proposed solution in a story, aimed at taking the audience through the journey of why the end results matter, how those results were achieved, and what to do about it.
Data science research does not often end in a well-crafted story, so creating engaging material for an audience can be challenging. Through borrowing the principles of storytelling used in design thinking, a few suggestions are as follows:
- Focus on explanatory analysis over exploratory analysis:Explanatory analysis presents an important finding or recommendation first, then explains the process that was taken to get there. Findings that are merely interesting and not useful are saved for in-depth descriptions of the project, or not included at all.
- Use visualizations with purpose: Start a visualization with writing out what needs to be communicated, then create exactly that. Often it’s easier to create a set of charts and graphs, then pull insights and craft a story around what has been created. This results in less-compelling visualizations. Instead, start with the purpose.
- Document the process as a journey: Sharing the steps that were taken to reach a conclusion helps an audience develop a deeper understanding of the final recommendations and inspire action. Use the journey of the research to create credibility and get buy-in from important stakeholders.
Potential Implications
The beginning of this paper discussed the expectations and search for the “unicorn data scientist” as one who excels in the technical, business strategy, and communication aspects of data science. Furthermore, the focus on the technical training associated with data science has left many lacking the strategic and communication skill sets.
The proposed Design Thinking Mindset for Data Science has the potential to assist technically-minded folks with the other aspects of the process, including framing the problem, expanding ideation through creative methods, performing exploratory analysis with the end goal in mind, gathering feedback on prototypes to keep stakeholders involved, and packaging the end results into a compelling story. Furthermore, as the technical components of data science progress further and further towards automation with AI-based data analysis products such as Watson, these identified aspects of data science work will becoming increasingly important as they are inherently human-centered and more difficult to automate.
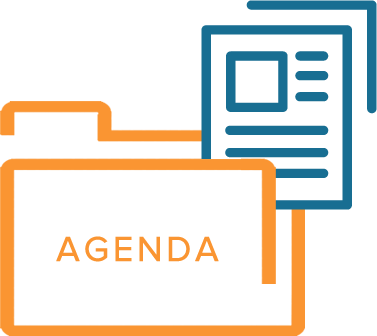

HTML


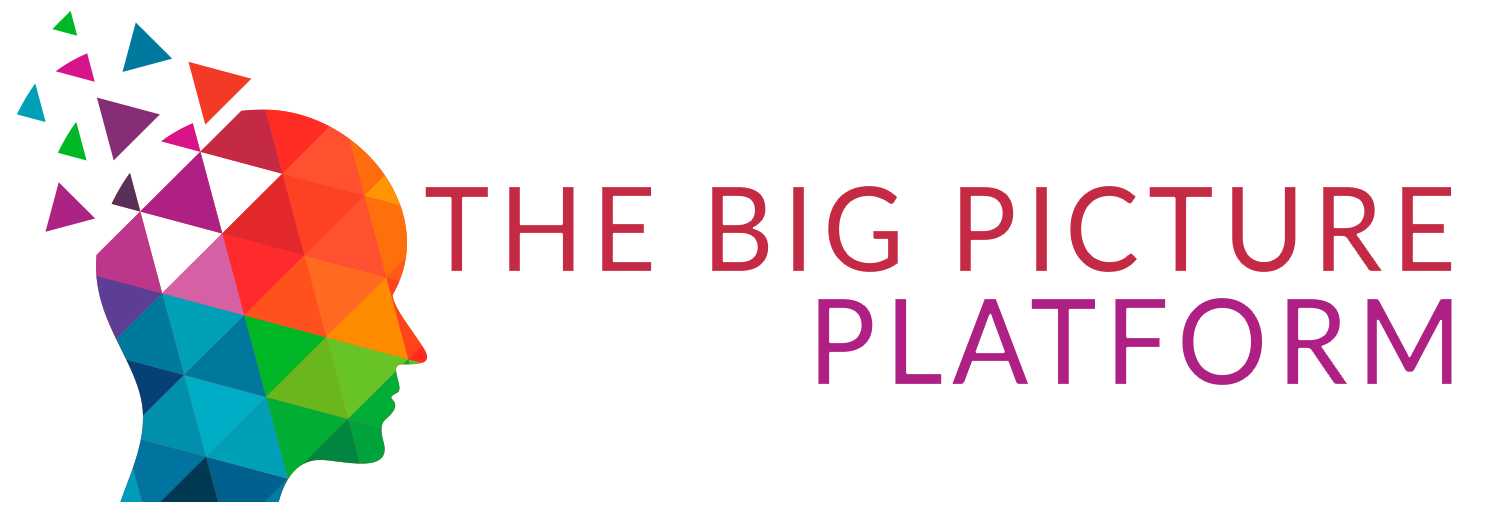
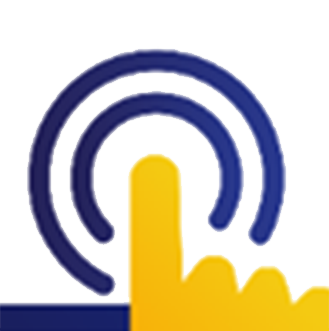
Objectives and Study Scope
This study has assimilated knowledge and insight from business and subject-matter experts, and from a broad spectrum of market initiatives. Building on this research, the objectives of this market research report is to provide actionable intelligence on opportunities alongside the market size of various segments, as well as fact-based information on key factors influencing the market- growth drivers, industry-specific challenges and other critical issues in terms of detailed analysis and impact.
The report in its entirety provides a comprehensive overview of the current global condition, as well as notable opportunities and challenges.
The analysis reflects market size, latest trends, growth drivers, threats, opportunities, as well as key market segments. The study addresses market dynamics in several geographic segments along with market analysis for the current market environment and future scenario over the forecast period.
The report also segments the market into various categories based on the product, end user, application, type, and region.
The report also studies various growth drivers and restraints impacting the market, plus a comprehensive market and vendor landscape in addition to a SWOT analysis of the key players.
This analysis also examines the competitive landscape within each market. Market factors are assessed by examining barriers to entry and market opportunities. Strategies adopted by key players including recent developments, new product launches, merger and acquisitions, and other insightful updates are provided.
Research Process & Methodology

We leverage extensive primary research, our contact database, knowledge of companies and industry relationships, patent and academic journal searches, and Institutes and University associate links to frame a strong visibility in the markets and technologies we cover.
We draw on available data sources and methods to profile developments. We use computerised data mining methods and analytical techniques, including cluster and regression modelling, to identify patterns from publicly available online information on enterprise web sites.
Historical, qualitative and quantitative information is obtained principally from confidential and proprietary sources, professional network, annual reports, investor relationship presentations, and expert interviews, about key factors, such as recent trends in industry performance and identify factors underlying those trends - drivers, restraints, opportunities, and challenges influencing the growth of the market, for both, the supply and demand sides.
In addition to our own desk research, various secondary sources, such as Hoovers, Dun & Bradstreet, Bloomberg BusinessWeek, Statista, are referred to identify key players in the industry, supply chain and market size, percentage shares, splits, and breakdowns into segments and subsegments with respect to individual growth trends, prospects, and contribution to the total market.
Research Portfolio Sources:
Global Business Reviews, Research Papers, Commentary & Strategy Reports
M&A and Risk Management | Regulation
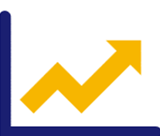
The future outlook “forecast” is based on a set of statistical methods such as regression analysis, industry specific drivers as well as analyst evaluations, as well as analysis of the trends that influence economic outcomes and business decision making.
The Global Economic Model is covering the political environment, the macroeconomic environment, market opportunities, policy towards free enterprise and competition, policy towards foreign investment, foreign trade and exchange controls, taxes,
financing, the labour market and infrastructure.
We aim update our market forecast to include the latest market developments and trends.
Review of independent forecasts for the main macroeconomic variables by the following organizations provide a holistic overview of the range of alternative opinions:
As a result, the reported forecasts derive from different forecasters and may not represent the view of any one forecaster over the whole of the forecast period. These projections provide an indication of what is, in our view most likely to happen, not what it will definitely happen.
Short- and medium-term forecasts are based on a “demand-side” forecasting framework, under the assumption that supply adjusts to meet demand either directly through changes in output or through the depletion of inventories.
Long-term projections rely on a supply-side framework, in which output is determined by the availability of labour and capital equipment and the growth in productivity.
Long-term growth prospects, are impacted by factors including the workforce capabilities, the openness of the economy to trade, the legal framework, fiscal policy, the degree of government regulation.
Direct contribution to GDP
The method for calculating the direct contribution of an industry to GDP, is to measure its ‘gross value added’ (GVA); that is, to calculate the difference between the industry’s total pretax revenue and its total boughtin costs (costs excluding wages and salaries).
Forecasts of GDP growth: GDP = CN+IN+GS+NEX
GDP growth estimates take into account:

Market Quantification
All relevant markets are quantified utilizing revenue figures for the forecast period. The Compound Annual Growth Rate (CAGR) within each segment is used to measure growth and to extrapolate data when figures are not publicly available.
Revenues
Our market segments reflect major categories and subcategories of the global market, followed by an analysis of statistical data covering national spending and international trade relations and patterns. Market values reflect revenues paid by the final customer / end user to vendors and service providers either directly or through distribution channels, excluding VAT. Local currencies are converted to USD using the yearly average exchange rates of local currencies to the USD for the respective year as provided by the IMF World Economic Outlook Database.
Industry Life Cycle Market Phase
Market phase is determined using factors in the Industry Life Cycle model. The adapted market phase definitions are as follows:
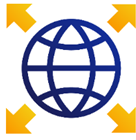
The Global Economic Model
The Global Economic Model brings together macroeconomic and sectoral forecasts for quantifying the key relationships.
The model is a hybrid statistical model that uses macroeconomic variables and inter-industry linkages to forecast sectoral output. The model is used to forecast not just output, but prices, wages, employment and investment. The principal variables driving the industry model are the components of final demand, which directly or indirectly determine the demand facing each industry. However, other macroeconomic assumptions — in particular exchange rates, as well as world commodity prices — also enter into the equation, as well as other industry specific factors that have been or are expected to impact.
Forecasts of GDP growth per capita based on these factors can then be combined with demographic projections to give forecasts for overall GDP growth.
Wherever possible, publicly available data from official sources are used for the latest available year. Qualitative indicators are normalised (on the basis of: Normalised x = (x - Min(x)) / (Max(x) - Min(x)) where Min(x) and Max(x) are, the lowest and highest values for any given indicator respectively) and then aggregated across categories to enable an overall comparison. The normalised value is then transformed into a positive number on a scale of 0 to 100. The weighting assigned to each indicator can be changed to reflect different assumptions about their relative importance.

The principal explanatory variable in each industry’s output equation is the Total Demand variable, encompassing exogenous macroeconomic assumptions, consumer spending and investment, and intermediate demand for goods and services by sectors of the economy for use as inputs in the production of their own goods and services.
Elasticities
Elasticity measures the response of one economic variable to a change in another economic variable, whether the good or service is demanded as an input into a final product or whether it is the final product, and provides insight into the proportional impact of different economic actions and policy decisions.
Demand elasticities measure the change in the quantity demanded of a particular good or service as a result of changes to other economic variables, such as its own price, the price of competing or complementary goods and services, income levels, taxes.
Demand elasticities can be influenced by several factors. Each of these factors, along with the specific characteristics of the product, will interact to determine its overall responsiveness of demand to changes in prices and incomes.
The individual characteristics of a good or service will have an impact, but there are also a number of general factors that will typically affect the sensitivity of demand, such as the availability of substitutes, whereby the elasticity is typically higher the greater the number of available substitutes, as consumers can easily switch between different products.
The degree of necessity. Luxury products and habit forming ones, typically have a higher elasticity.
Proportion of the budget consumed by the item. Products that consume a large portion of the
consumer’s budget tend to have greater elasticity.
Elasticities tend to be greater over the long run because consumers have more time to adjust their behaviour.
Finally, if the product or service is an input into a final product then the price elasticity will depend on the price elasticity of the final product, its cost share in the production costs, and the availability of substitutes for that good or service.
Prices
Prices are also forecast using an input-output framework. Input costs have two components; labour costs are driven by wages, while intermediate costs are computed as an input-output weighted aggregate of input sectors’ prices. Employment is a function of output and real sectoral wages, that are forecast as a function of whole economy growth in wages. Investment is forecast as a function of output and aggregate level business investment.